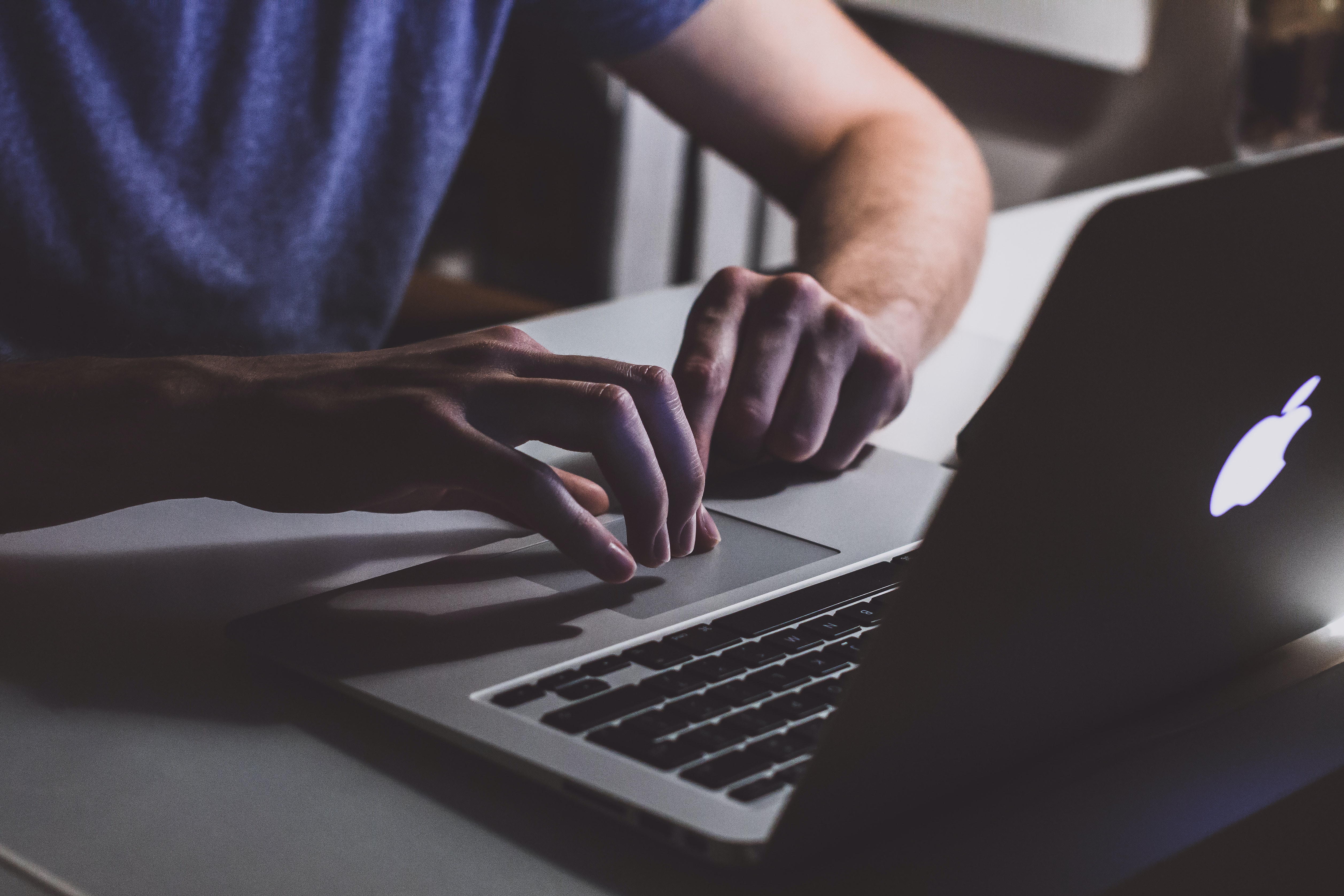
Multilevel (i.e., hierarchical, nested) data is common within behavioral health research. Examples include data from patients nested within doctors and children nested within childcare centers. Longitudinal data also has a hierarchical structure, where repeated measurements can be viewed as nested within individuals. The nested structure of the data allows for a wide range of potential research questions to be addressed. For example, the influence of both patient-level (e.g., age) and doctor-level (e.g., years of experience) variables on patient health outcomes can be simultaneously modeled. Despite the utility of hierarchically structured data, the analysis of such data is often more complex than the analysis of data without a hierarchical structure and specialized software is often needed. A major aim of my research is to develop novel methods for the analysis of multilevel data and implement these methods within easy-to-use software packages so that they are accessible to a broad range of applied researchers.
One of the software programs I have developed is MLMED (Rockwood, 2017), an SPSS add-on that can be used to fit multilevel mediation and moderated mediation models. Mediation and moderation analyses allow researchers to better understand how causal effects operate and the conditional nature of such effects (Hayes & Rockwood, 2017). For example, suppose a researcher is interested in the effectiveness of a new substance abuse intervention. Mediation analysis can be used to determine how or why the intervention is effective. Perhaps the intervention reduces substance craving which, in turn, reduces substance use. Moderation analysis, on the other hand, can be used to determine for whom or under what conditions the intervention is effective. Perhaps the intervention is more effective for users of a certain class of substances, but not users of other substances. Or maybe the effectiveness of the intervention depends on the social support system of the individual. Although it is possible to estimate such types of effects using multilevel data within SPSS without MLMED, doing so requires a large amount of data management and leaves much room for error. MLMED, on the other hand, drastically simplifies the process and requires only a single line of code. So far, MLMED has been used in over 75 publications by researchers in over 50 countries.
My research also focuses on developing methods and software for general latent variable modeling, a broad class of statistical models that includes multilevel models, structural equation models (SEMs), and item response theory (IRT). I helped create SPIRIT, an SPSS macro for explanatory IRT analyses, and used the developed software to model children’s ADHD symptoms (DiTrapani, Rockwood, & Jeon, 2018). I also co-developed PLmixed (Rockwood & Jeon, 2019), an R package for complex measurement and growth modeling. PLmixed is particularly suited for modeling data with multiple hierarchical structures, such as longitudinal data from students during their transition from middle school to high school. My current research is focused on the development and implementation of computationally efficient estimation methods for multilevel structural equation modeling (MSEM), a flexible statistical framework for the analysis of multilevel data. I am in the process of implementing an estimation algorithm I developed (Rockwood, in press) into a popular SEM package in the R computing environment and will also be working towards expanding the methodology to a wider range of response variables.
References
- DiTrapani, J., Rockwood, N. J., & Jeon, M. (2018). Explanatory IRT analysis using the SPIRIT macro in SPSS. The Quantitative Methods for Psychology, 14(2), 81–98.
- Hayes, A. F. & Rockwood, N. J. (2017). Regression-based statistical mediation and moderation analysis in clinical research: Observations, recommendations, and implementation. Behaviour Research and Therapy, 98, 39-57.
- Rockwood, N. J. (in press). Maximum likelihood estimation of multilevel structural equation models with random slopes for latent covariates. Psychometrika.
- Rockwood, N. J. (2017). MLmed: An SPSS macro for fitting multilevel mediation and moderated mediation models. https://njrockwood.com/mlmed.
- Rockwood, N. J. & Jeon, M. (2019). Estimating complex measurement and growth models using the R package PLmixed. Multivariate Behavioral Research, 54(2), 288–306.